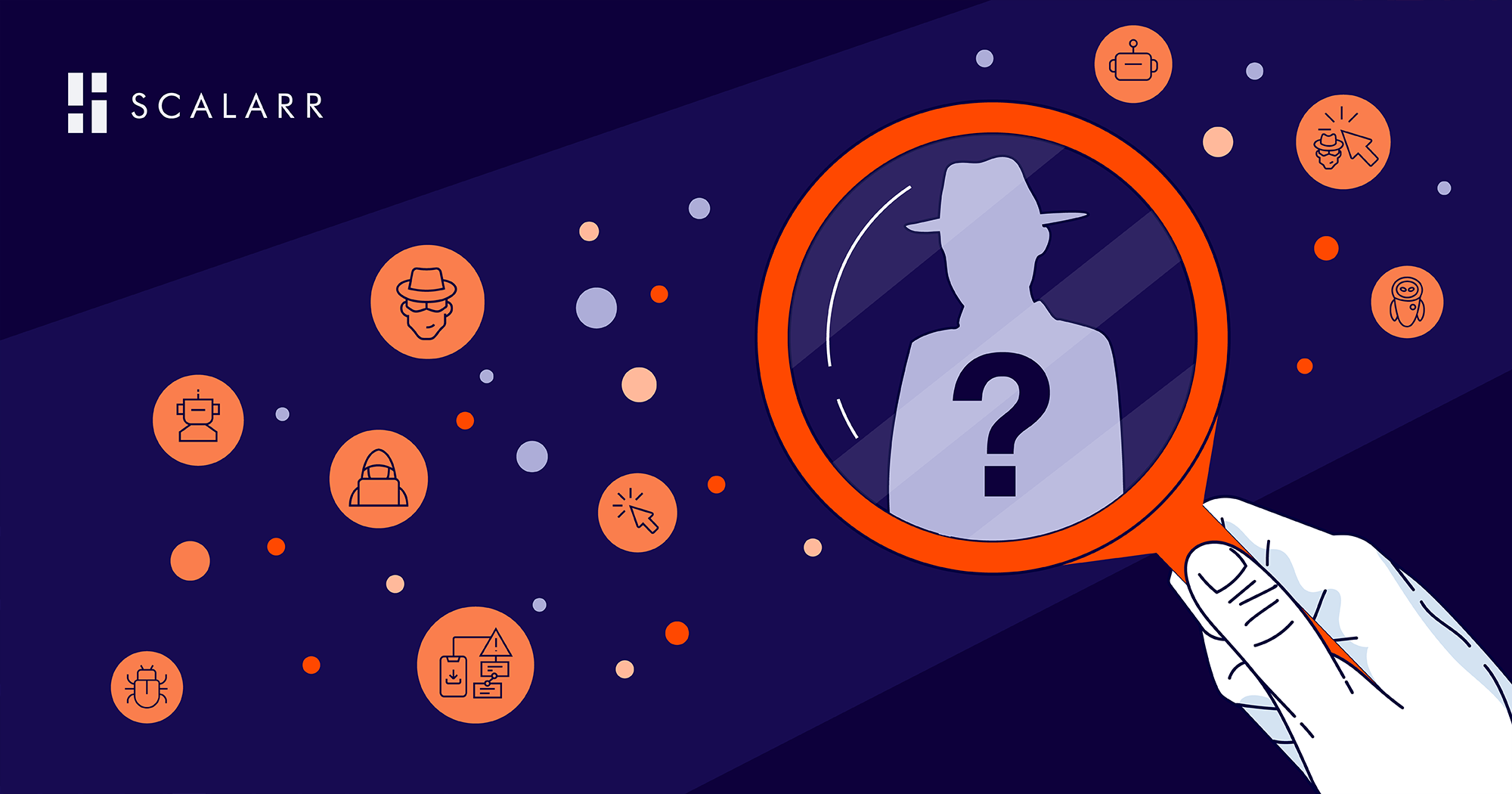
The truth about mixed fraud
Mobile ad fraud has always been a headache for mobile app advertisers across the globe, but the pandemic has evidenced even more how vulnerable the entire mobile ecosystem actually is. This is due to the sheer amount of time users spend on their mobile devices and how much new content they’re demanding. It’s unprecedented and nothing anyone could have predicted or prepared for.
Entire workloads moved to mobile apps, be it for educational purposes, government services, entertainment, shopping, or news, everyone was on their mobile devices trying to stay connected with the world. It’s safe to say that the past 24 months have arguably been one of the most uncertain and turbulent times for mobile app advertisers, many of whom had to endure slashed budgets and suspended campaigns, all while having to keep up with the mounting pressure of servicing end users and keep everyone connected.
Unfortunately, fraudsters translated this uncertainty into an opportunity to scale their operations, taking advantage of the fact that people were spending more and more time on their mobile devices, which is why we’ve seen a surge in malware activity and a higher degree of sophistication in their fraud attacks.
With new evolving types of fraud floating around, and billions of dollars at stake, mobile app advertisers need to strengthen their walls of defense against fraud tactics that are draining their advertising resources. While some of those fraud types can be familiar and relatively widespread like click injection, click spam, or bots, there are other more refined fraud types that are more problematic to identify including modified click spam, smart bots, complex VTA spoofing, or mixed fraud.
For today’s article, we’ll focus on mixed fraud or fraud mixes, which is deemed as the most advanced type of scam as of yet.
What does mixed fraud entail?
Mixed fraud usually consists of different fraud types mixed together in one indivisible cluster. This type of fraud can be packed in more insidious variations under the ruse of disguising fraudulent traffic in clean traffic.
On principle, the ground for mixed fraud is the conscious use of several types of fraud to bypass known or traditional protection measures of fraud detection tools. In other instances, it can also be a chaotic mixture of different fraud types if connected to the publisher with an uncontrolled number of re-brokering levels.
Mixed fraud inside a cohort can be identified in the following combinations:
- One type of fraud and non-fraudulent traffic
- Several types of fraud
- Several types of fraud and non-fraudulent traffic
The main problem with identifying mixed fraud types is that almost all anti-fraud solutions enforce rules-based detection and analysis of cohorts within an undivided bundle. As such, all rules and metrics of almost all anti-fraud solutions are available only at this level of granular detail, which is not efficient enough in detecting mixed fraud. An example of a mixed bundle can be: app → publisher → sub-publisher → sub-sub-publisher → campaign.
This type of fraud is very hard to detect because there are no obvious patterns that can be easily recognized and interpreted as fraudulent traffic. There is still a lot of work to be done in the anti-fraud industry to be fully equipped against this type of fraud.
Thankfully, mixed fraud can be identified by Machine Learning algorithms. In light of the growing threat that mixed fraud poses for the mobile advertising industry, it’s important to take conscious steps towards fighting this specific type of fraud, as Scalarr traffic analysis shows that mixed fraud accounts for up to 16% of all fraudulent installs.
Real-life mixed fraud examples
The most popular combinations of mixes include smart bots + click injection, smart bots + modified click spam, and smart bots + modified click spam + real users.
Despite the complexity of properly identifying mixed fraud, one of the most effective ways to protect advertising campaigns is to leverage traffic clustering technology. This type of technology relies on a Machine Learning algorithm that isolates individual fraudulent clusters inside the indivisible bundle.
Below, you can see a review of mixes from short real-life examples of two of the most popular and attractive app categories for fraudsters: games and e-commerce.
- A popular mobile MMORPG game. Fraudsters disguised under two types of fraud, smart bots and modified click spam. The idea behind their attack is to program smart bots with significantly short time-to-install (TTI). By doing so, fraudsters created a TTI distribution similar to what it would organically look like, when in reality it was a mix of smart bots and click spam. In this situation, a developer without advanced anti-fraud protection would have considered this bundle as normal and qualitative. To add even more confusion to the mix, this type of mixed fraud is intentionally divided into small bundles. Each of these clusters was successfully identified thanks to the use of unsupervised learning by applying clustering models proprietary to Scalarr. As a result, the mobile game developer was able to see the fraudulent attack, reject the fake installs, and save money.
- An e-commerce shopping app. Here, fraudsters attempted to mix click injection with non-fraudulent traffic. The premise of such an attack lies in the fact that this specific type of mix makes the overall traffic look non-fraudulent. Without fully-labeled data sets to go by, Scalarr leverage new data points from Google Play including referrer time, install time, and more, for further traffic clustering. This approach enabled us to have fully isolated fraudulent clusters inside the indivisible bundle and clearly make the distinction between fraudulent and non-fraudulent traffic.
Conclusion
As of today, mixed fraud is one of the most problematic fraud types in terms of identification. With low performance and effectiveness from rules-based solutions, it’s clear that only traffic clustering technology based on advanced Machine Learning algorithms can allow for the accurate detection of fraudulent and non-fraudulent traffic, as well as the different types of fraud used.
The only solution for mobile advertisers is to partner with anti-fraud tools that make use of powerful and intelligent technologies such as machine...
The road to Scalarr's foundation was paved with challenges and opportunities and in this in-depth conversation, you'll learn the story of Scalarr f...