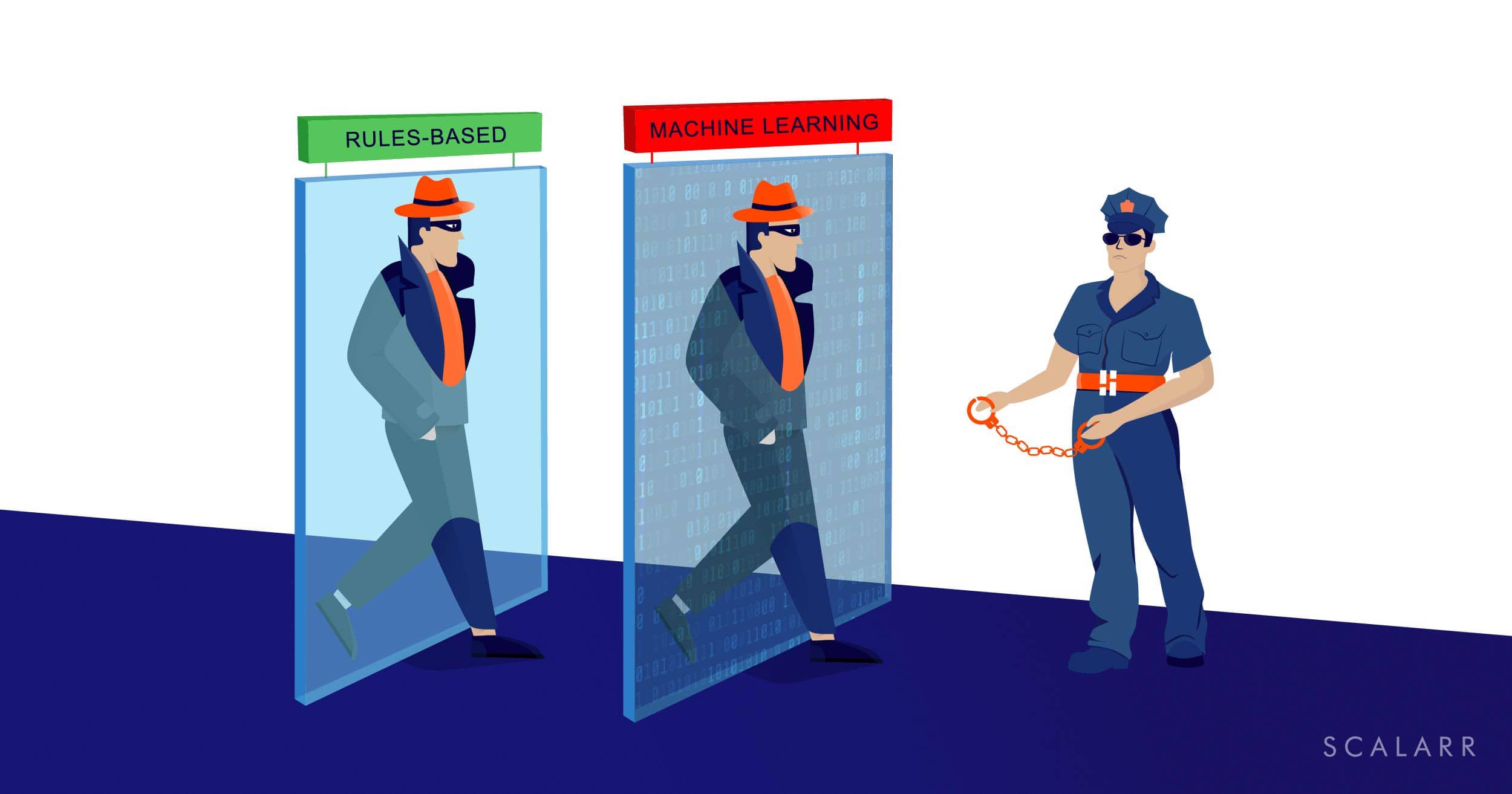
Fraud is a big enough problem that requires an all-hands-on-deck approach. With estimated losses circling the $12.6 billion mark by the end of 2019, it’s no wonder that app developers are on the search for the optimal anti-fraud solution.
As the popularity of mobile apps rises, with no sign of stopping any time soon, new threats emerge from all fronts. In fact, one of the latest trends in mobile advertising is the speed with which fraudsters reverse-engineer their criminalistic attempts to remain undetected and continue to decimate marketing budgets.
So, what’s the best protective measure that app developers can take to safeguard an app?
The best protection and the one that offers more coverage is to choose a 3rd party anti-fraud solution partner who can alleviate the processing of daily traffic and solely focus on discovering abnormalities and hidden fraud patterns.
Having said that, the selection of the best anti-fraud solution partner is a hefty task that requires an understanding of the technology they employ and how they operate. Overall, 3rd party solutions can be divided into two groups: rules-based and machine learning-based solutions.
Anti-Fraud Solutions and How They Work
Let's kick off with rules-based solutions. For some time, these were the preferred choice for most advertisers as the options were very limited. Rules-based solutions employ techniques that identify when key characteristics and events exceed or fall below specific parameters.
But the catch is that these solutions only perform well when you know every single thing that plays a role in a specific type of fraud. There is a lot of observation that goes into creating a new rule since the rule must be thoroughly instructed to recognize very specific scenarios so it can confidently discern valid traffic from fraudulent traffic. And the issue with this type of approach is the high percentage of false positives/negatives it can lead to.
On top of all this, rules-based solutions are very easy to reverse-engineer by fraudsters given the fact that all rules are exhaustively predefined and they do not self-learn, making it extremely difficult to find new or smart fraud types.
For years now, many believed that a traditional solution provided enough coverage and protection against fraud - and to this day, unfortunately, many app developers and marketers still operate under the illusion that by keeping fraud levels low with the use of a rules-based solution, they are protected.
But the reality is that nowadays, fraud has become much more complex and sophisticated making it virtually impossible for traditional, rules-based solutions to meet the expectations and the level of protection that app developers require to fully safeguard their product.
One of the best approaches is to use intelligent technologies such as machine learning. Machine learning is a notably powerful tool on its own, but it's even better when it's coupled with deep expertise, continuous development of the technology itself, and different combinations of algorithms.
With that being said, app developers should push for machine learning-based fraud detection to ensure the full-blown protection of their app. Why?
Because machine learning can process colossal amounts of data without hindering performance or quality in outcomes.
Inherently, machine learning provides a consistent, reliable, scalable, and automated setting where machine learning algorithms thrive as they process every piece of data fed into the engine.
Overall, the advantages that machine learning holds over rules-based solutions in terms of fraud detection are:
- Machine learning algorithms can recognize hidden fraud patterns and make associations based on data from post-install activity.
- It has the ability to process billions (and more) of data points simultaneously, and what’s more, it self-trains which leads to a dramatic increase in accuracy when it comes to fraud detection.
- The unsupervised machine learning engine can work with unstructured data that does not need to be labeled and automatically discover and learn patterns from a massive amount of data by specifically looking for abnormalities and their underlying connections.
- Inherently, machine learning collects experience and learns from it.
Together, these clear advantages bring about the maximum level of completeness in fraud detection (the ability to detect all types of fraud) and the maximum level of accuracy (minimum number of false positive and false negative results). That's why prepared and highly analytical advertisers and marketers should favor machine learning anti-fraud solutions as the instrumental component in the fight against fraud.
Note:
Machine learning-powered engine is able to provide the maximum level of completeness in fraud detection and the maximum level of accuracy.
Take the case of TextNow, a Scalarr client who was unknowingly the target of fraud in ad views. By performing an in-depth daily analysis of TextNow data, Scalarr's ML-based anti-fraud engine was able to process, analyze, and ultimately detect the presence of fraud by using a powerful machine learning engine that is capable of unveiling every abnormality in data. As a result, a new type of fraud was discovered (Complex VTA Spoofing) and the client was spared from losing tens of thousands of dollars a month in media spend.
On the contrary, machine learning becomes an automated process that requires no supervision in the detection of abnormalities, simplifying the unveiling of hidden changes in patterns and behavior of data.
This is particularly important as there are subtle, barely perceptible changes in data that may not be overtly evident to the human eye, but can signal fraud for machine learning algorithms. You see, with machine learning, the possibilities are endless as anti-fraud solutions can create algorithms that process any amount of data with as many variables as needed to help find these hidden abnormalities and call out the presence of fraudulent activity.
To sum up
For some years now, machine learning has been spearheading the most accurate, conclusive, and powerful fight against fraud and the results are positive, with more fraud types being addressed and detected thanks to this cutting-edge technology. So now, the only question that remains is: are you using the latest and greatest in technology to fight fraud?
Hesitate no more and make machine learning anti-fraud solutions a part of your strategy to fight fraud straight away.
***
If you want more details about how Scalarr’s anti-fraud tool can employ personalized machine learning models specifically for your app and that guarantee accuracy of up to 97% in fraud detection, request a trial and contact us so we can work together.
The only solution for mobile advertisers is to partner with anti-fraud tools that make use of powerful and intelligent technologies such as machine...
The road to Scalarr's foundation was paved with challenges and opportunities and in this in-depth conversation, you'll learn the story of Scalarr f...