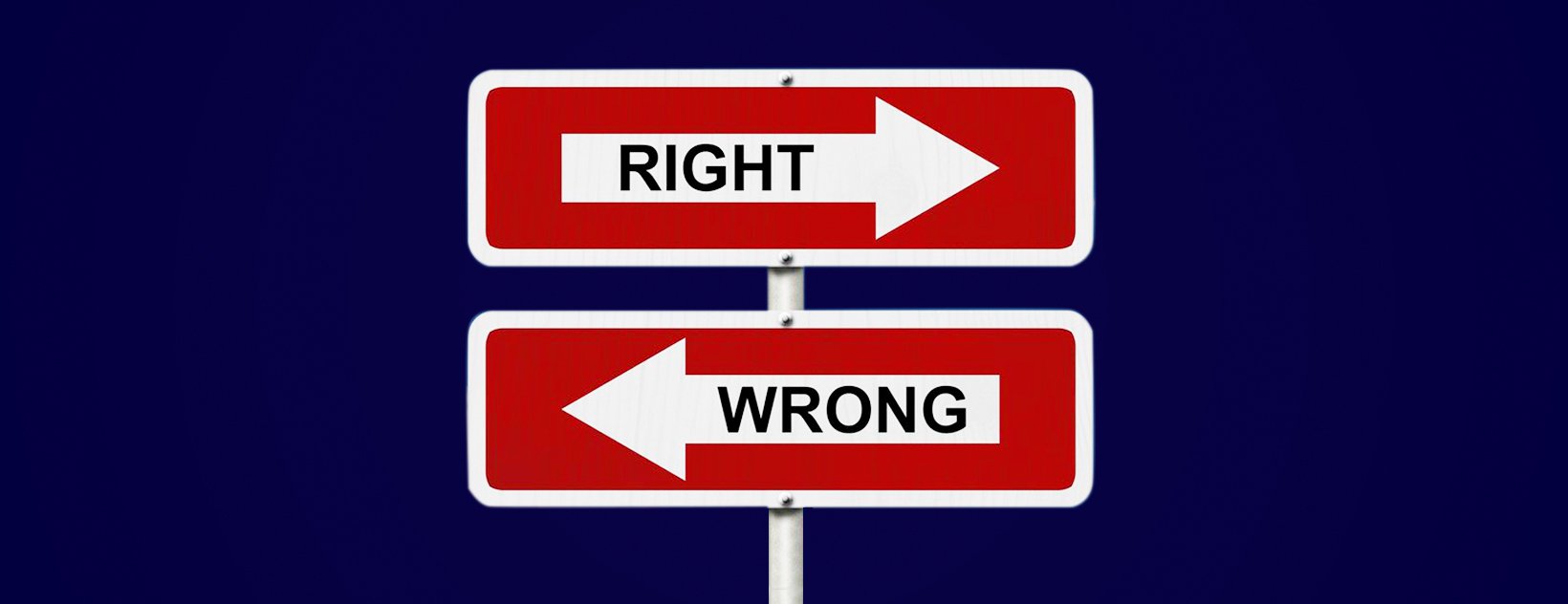
Today's the most sophisticated threat landscape is mobile app install market. Mobile app install fraud was a hot topic one year ago and still is the same, being that thing that moves user acquisition the wrong way. For $14bn market fraudsters will cause $3.6bn loss in 2017.
Within the same trusted network, you can have campaigns with fraud rates below 3% and other ones above 95%. It is very interesting that fraud rates vary from country to country.
The fraud rates are bigger for the high LTV markets, including such countries as the US, Germany, the UK, Japan and South Korea. Android devices are more vulnerable, still often you can see the comparable fraud rates for both Android and iOS within the same campaign.
The biggest challenge mobile advertisers need to face is that the mobile app install fraud has revolutionized within the year and become hardly detectable, as well as new smarter types erased. It is very difficult for traditional anti-fraud solution to detect the latter.
The most widespread fraud types:
1. Attribution Fraud Category includes such types as the classic click-spamming, modified click-spamming, click injection. Their main goal is to ‘steal’ the organic traffic and that one from other paid sources. Unfortunately, “last-action” attribution used in app-install tracking only helps fraudsters here.
2. Sophisticated Bots. Comparing to simple bots sophisticated ones fully emulate the user behavior by doing all post-install activities for a long time. From human perspective they are almost alike real users having the personal IP, device ID, etcetera. Sophisticated bots are very hardly detectable. This is an advanced type of fraud.
3. Financial Fraud is often recognized as a part of sophisticated bots fraud in order to ‘show’ a user acquisition manager that the traffic is ‘real’ and make him scale the source. A lot of payments are made, but the majority of them are fake and done from stolen cards.
4. ‘Mixes’. That is a totally new and very dangerous type of fraud, as here we can observe different types of fraud, as well as real users are mixed within one sub-publisher. E.g. Real users and fraudulent ‘fake’ installs, or real users and different types of fraud, such as attribution fraud and bots.
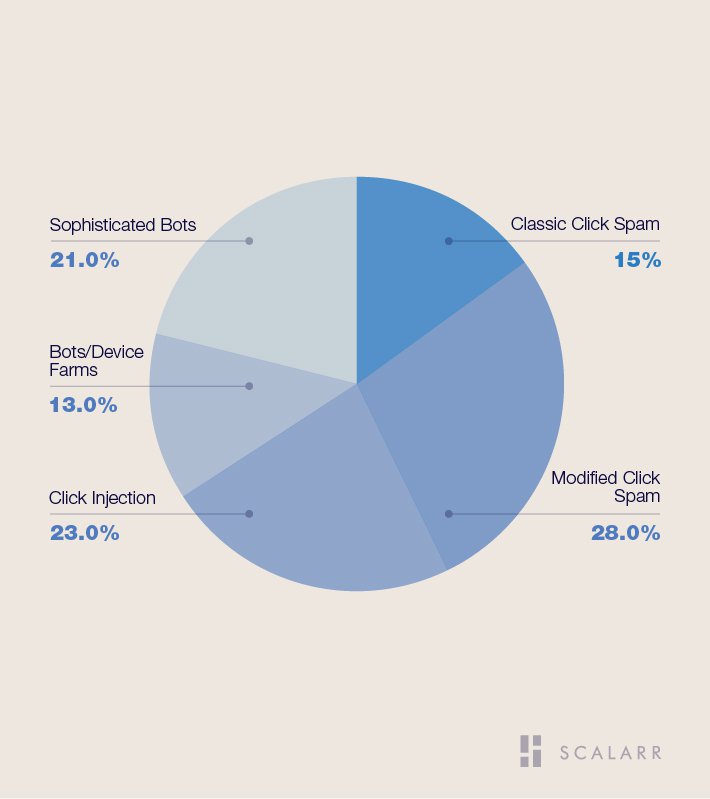
Still there are other fraud types, including incentive injections, emulators, device farms and even more. Their percentage is lower, but here the principles of long tail should be applied to. In overall, they have a large number of occurrences increasing the stake.
The most important thing that the mobile advertisers should consider that fraudsters are advanced technology-based companies, whose main goal is to not be caught. They put all efforts to succeed, and if the fraudulent traffic is not stopped or rejected in time, they will definitely scale. The approach for detecting the fraud should be changed dramatically and become more advanced.
Dark side accomplishes its algorithms
Let’s see the examples we spotted recently.
One of the biggest type of attribution app-install fraud is the click-spam (or ad stacking, or click flooding). We observe how fast the fraudsters adjust their algorithms making them literally undetectable for traditional rules-based analysis.
Thus, two sub-types of click-spam should be emphasized. These are: the classic click-spamming and modified one. The pattern of the first sub-type has an abnormal time-to-install distribution within seven days and is characterized by having a large number of installs distributed randomly far from the ‘normal installs distribution’ in the very beginning.
Here are examples for the real users' distribution model and “classic click-spam” distribution model.
Keep in mind:
The most important thing that the mobile advertisers should consider that fraudsters are advanced technology-based companies, whose main goal is to not be caught. They put all efforts to succeed.
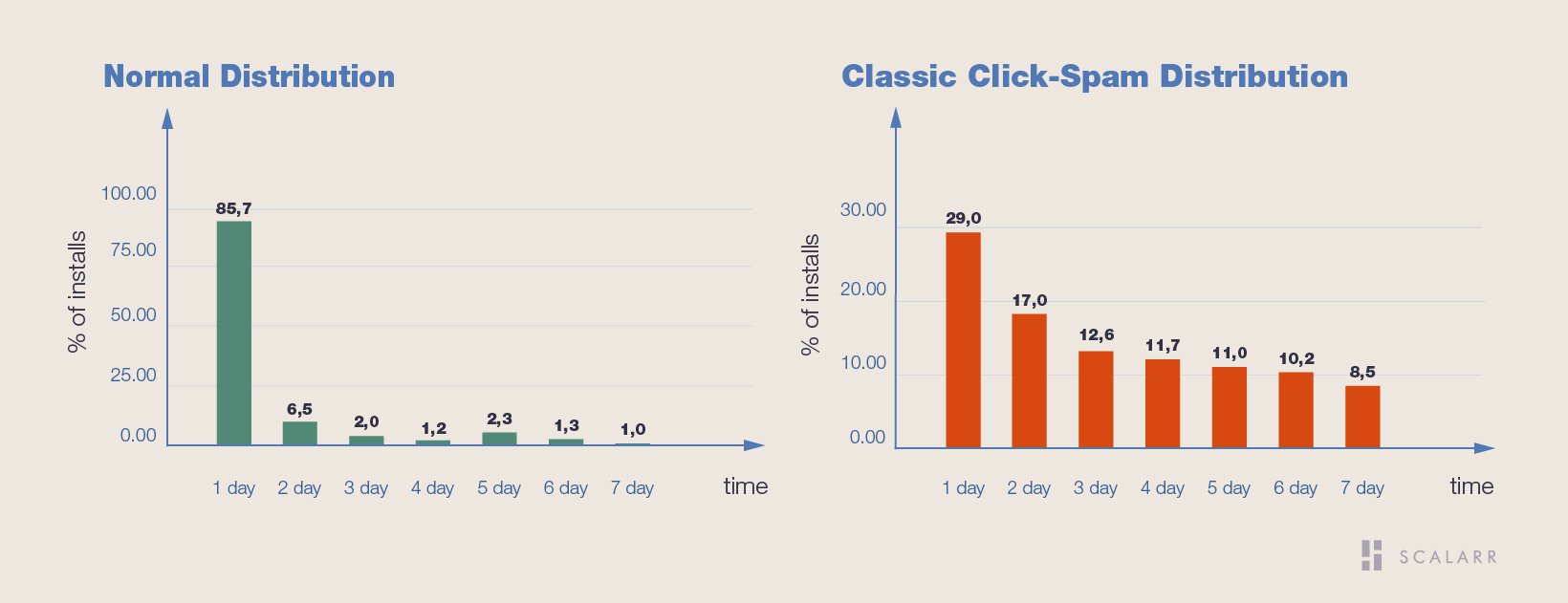
When click spammers receive the information or precise metrics in the form of guides or reject reports, they use them to modify the algorithm.
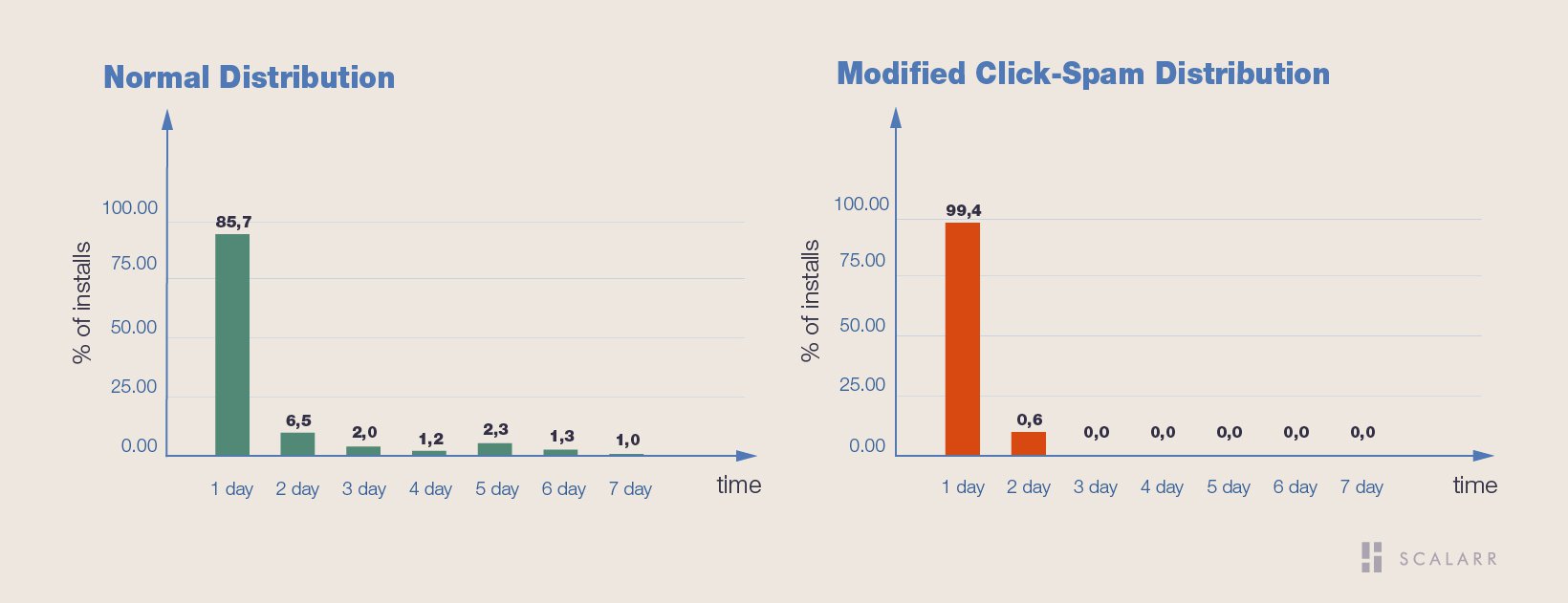
These two diagrams look very similar, aren’t they? But the second one still shows click spam. If taking a look at the installs hourly distribution, you can see the same click spam pattern.
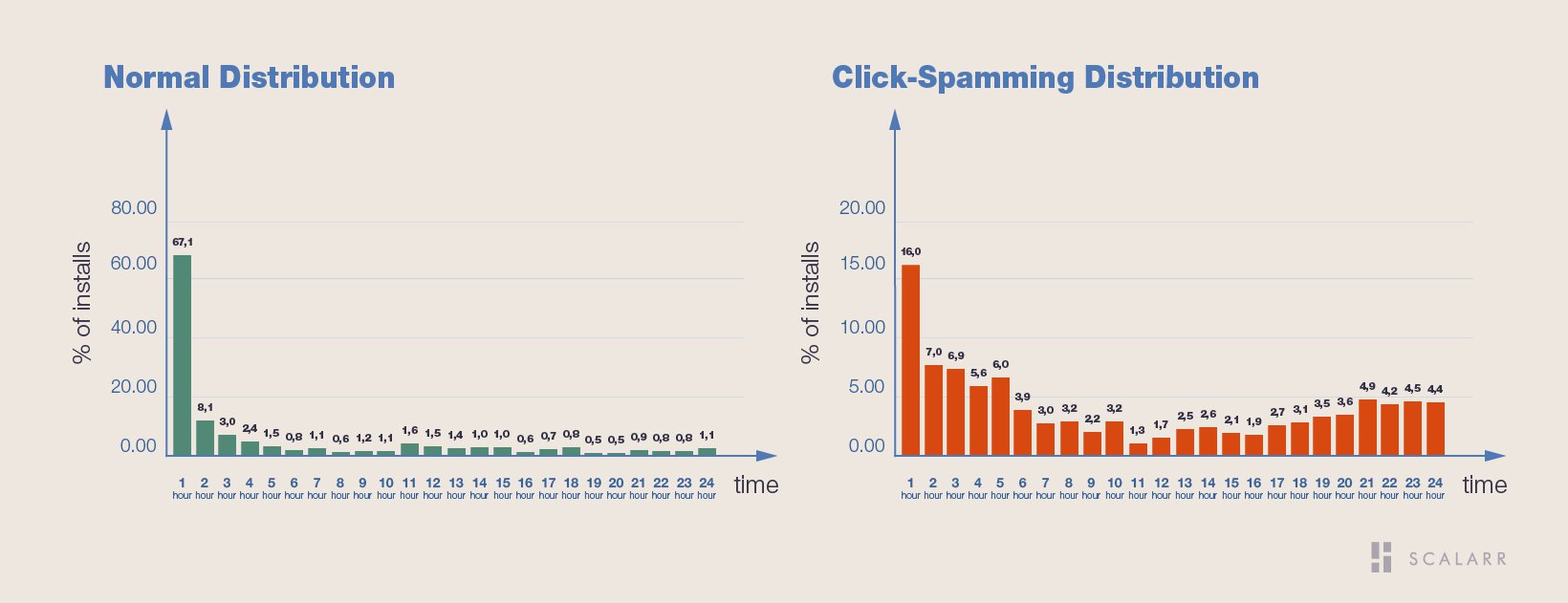
Unfortunately, the click spammers have received a detailed reject report once again, and their next step, of course, was to improve the existing algorithm even more.
While modifying their algorithms consistently, the fraudsters stay invisible for the automated rules-based analysis. True ML is capable to identify all these changes immediately. Take a look at these ‘clusters’:
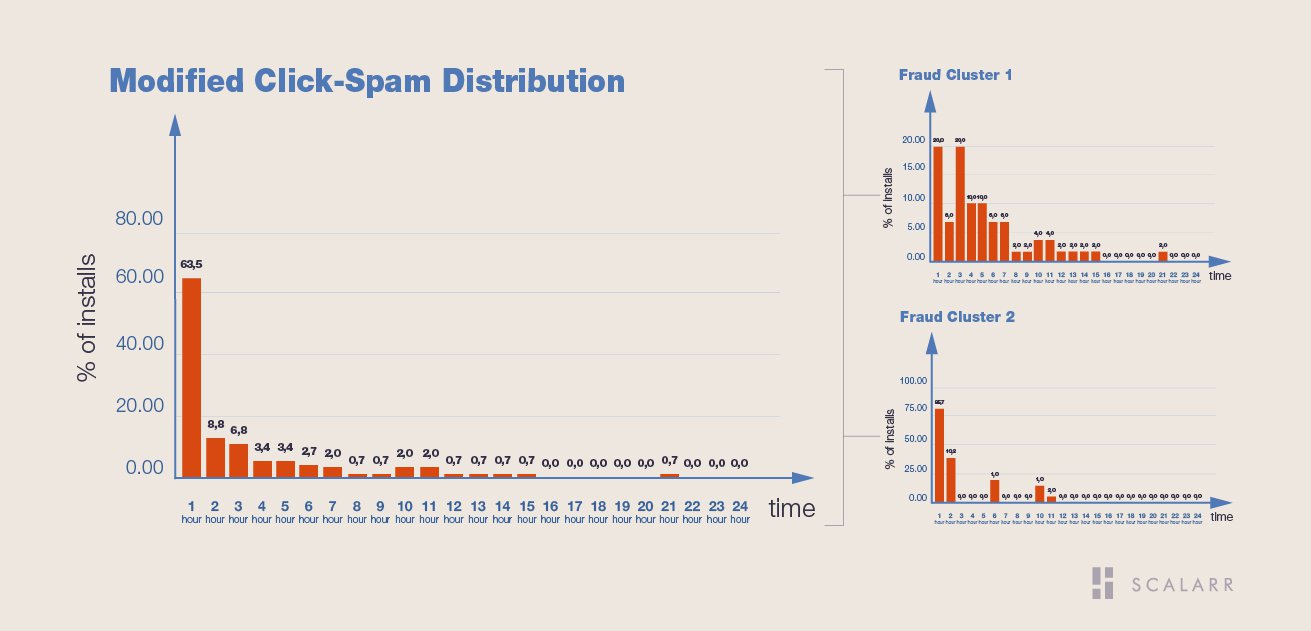
Of course, this is a simplified image of the ML algorithms in work.
Another good example of the fraudster’s fast reactions is the “% of new device” metrics. This metric could be very efficient in revealing some type of fraud. But the problem still remains the same. Fraudsters know this metric.
So within some time the app is flooded again with the unexplained increase in number of downloads without any further activity inside the product. One of the hypotheses which should be named is that all these devices aren’t more ‘new’, and the rule doesn’t work here anymore.
Let’s mention here ‘carousel effect’ too. When the fraudsters have modified and improved their algorithm or methods, they will try to ‘infect’ you again and again within the time. Very often they do that using other ‘sub-IDs’, while working with your biggest publishers, whom you scale consistently.
New approach
By aiming the steady growth, each developer wants to see increases pretty consistently across the markets. The same is about fraudsters. Among 100 installs bought at least 25 of them are fraudulent.
A commonly used approach of traditional anti-fraud solutions in detecting the app-install ad fraud is the rules-based analysis, as was mentioned before. Even with using buzzwords as “machine learning”, “big data”, “artificial intelligence” that are still rules, heuristics inside.
All fraud reasons and their specific meanings are available to clients (existing traditional anti-fraud solutions) via website / via dashboard / via numerous white-paper and presentations / via account managers (anti-fraud solutions).
They are also available to publishers and sub-publishers via the same channels, plus they receive the detailed rejections reports from user acquisition managers. Thus, the fraudsters can easily see it and use to reverse-engineer and re-work their strategy. That is why, in the majority of cases they new “attacks” succeed.
Unfortunately, the traditional rules-based analysis just isn’t efficient in detecting the new modified fraud patterns.
And, of course, the developer is unable to make a clear decision on this traffic too, as the pattern is already different, more advanced. Of course, in this situation UA team proceeds paying for the fraudulent traffic and very often scale these sources.
Only deep and machine learning technology backed solution can solve the problem. Machine learning analyses the dozens of features and a hundred of connections between these features, which are not seen or understandable to humans. That makes it flexible and capable to detect absolutely new types and patterns of fraud.
This methodology significantly increases the level of protection.
More issues to address immediately
Unfortunately, not all user acquisition managers understand the fraud and treat the issue accordingly. They benefit daily metrics and set the KPIs for the traffic basing on them.
But that helps the fraudsters a lot, as they need only to provide fake installs, which would meet the requirements. Even payments are made in order to prove ‘the traffic is real’.
Lack of understanding and knowledge leads to the wrong decisions. Actionable analysis shall include not only daily metrics, but also tracking different usage and financial metrics for far long period.
One more issue that is very disturbing and should be changed is actually the bonus systems of UA teams, which is oriented on getting as many installs as possible, not the quality ones.
That leads to the situation when the ‘fraud signals’ are often ignored, as they are just not interested in stopping or rejecting these sources.
How the developer address these issues will be the main factor determining whether the revenue growth continues as strongly in the next few months and years as it has in the recent past.
Originally published at www.pocketgamer.biz.
The only solution for mobile advertisers is to partner with anti-fraud tools that make use of powerful and intelligent technologies such as machine...
The road to Scalarr's foundation was paved with challenges and opportunities and in this in-depth conversation, you'll learn the story of Scalarr f...