App install fraud is a hot topic in the mobile industry for a few years already. This fact is confirmed by 78% of brand marketers being concerned about ad fraud and bot traffic (as was estimated last year, the economic losses due to bot fraud was around $6.5 billion globally in 2017, in particular app-install fraud losses was more than $3,6 billion). For apps advertisers, the issue of fraud is as up-to-date as ever. Fake installs affect on app metrics, forcing marketers to throw more and more money in a tube. Fraud is an adaptive crime, so it needs special methods of intelligent data analysis to detect and prevent it. Let’s see what is worth paying attention to in the fight against mobile ad fraud.
Anti-fraud solutions and their methods of working
The issue is evolving: fraud strategies develop and become more sophisticated. And the problem is that the most advanced types of fraud are on the rise. By Scalarr estimates, the share of “mixes” (the mixture of different types of fraud or mixture of real users and app-install fraud) increased by 118.7% from January to March of this year. We also discovered a lot of mimicry during this year, which is one of the most hardly detectable types of fraud. So now fighting with fraudulent activities without an appropriate anti-fraud solution becomes impossible. But choosing the right fraud detection solution with proper technology might be not so easy as it seems since the market is saturated by different anti-fraud services. With a detailed look at the market of the anti-fraud solutions becomes easy to divide all existing solutions into two groups depending on the technologies they use for mobile fraud detection: rules-based and ML-based anti-fraud solutions. Solutions from these two groups have significant differences in the way of identifying fraudulent activities:
1. Rules-based anti-fraud solutions are one-size-fits-all — they are trained to do what is programmed without adding intelligence. They follow a binary view of whether the rules criteria are met or not and are not able to detect new (unknown) and modified patterns of fraud. This results in more false positives or false negatives and low accuracy of fraud detection. While rules-based solutions serve their purpose in certain spheres, their ability to adapt to the modern world of big data is limited.
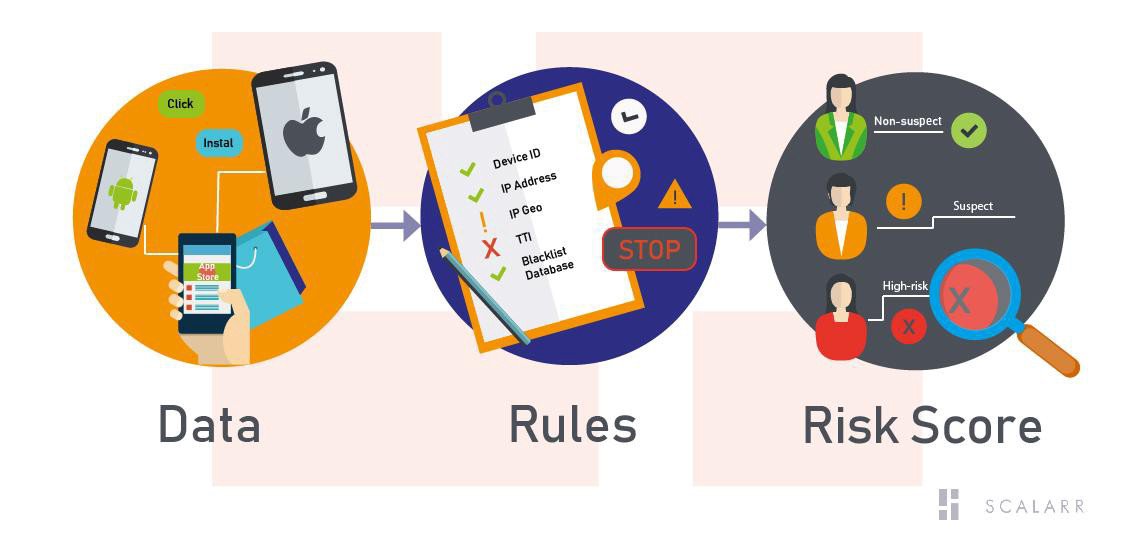
2. Machine Learning based anti-fraud solutions — machine learning ML algorithms automate the investigation of more data than is possible for a human, correlating thousands types of data points like time to install, IP address, domain, top cities, countries, devices, time to 1st transaction after sign-up, abnormal purchase patterns and so on. ML-based solutions are able to learn from the data just like a human learns from experience. As you get deeper into the topic of machine learning solutions, you can discover that they might be using different algorithms, which determine the way how they work and detect fraud installs. Broadly, machine learning algorithms can be classified into three types based on the way they “learn” about data to make decisions and predictions to fight mobile ad fraud: supervised (SML), semi-supervised (SSML) and unsupervised learning (UML).
Supervised learning named so because it needs to be given a set of predictors (independent variables). In other words, it has a guide in the person of data scientist, who teach the algorithm how it should operate and what conclusions it should output. Based on that, SML is showing good results at capturing already known types of fraud. But when it comes to new evolving types of fraud, the effectiveness of SML greatly decreases. On the other hand, unsupervised machine learning is already able to identify complex processes and patterns through clustering and segmenting input data in different groups for specific intervention without any target variable to predict or estimate. UML algorithms are self-learning. Such algorithm will be more effective for detecting new fraudulent activity, which is not already learned from existing dataset. However, despite the fact that UML gives the most accurate result, this result is very difficult to interpret to indicate the causes of fraud. For this issue perfectly suited Semi-Supervised ML algorithm that uses a small amount of labeled data with a large amount of unlabeled data. Using SSML in addition to UML can considerable improve the accuracy and completeness of the fraud identification and allows you to easily interpret the fraud reasons in a human-readable form. As a result, a well-rounded solution uses these both types of algorithms to build predictive data models that help companies to prevent any fraud threat.
In addition to differences between Rules-based and ML-based models we should say that rules-based systems, whose model is based on metrics, flag up to 50 percent of users as suspicious and requires additional review by a human. At this point, might be hard for user acquisition managers to distinguish all fraudulent traffic from non-fraudulent. Especially, considering the fact that from affiliate networks fraudsters actively started to seep into “trust”. The share of the fraud, which we can explore now in such sources varies from 2 to 14% averagely, but just a few years ago such numbers were impossible. On the other hand, machine learning solutions are able to give a univocal result whether it’s fraud or not a fraud. Below is the simplified working model of Scalarrs’ anti fraud solution:
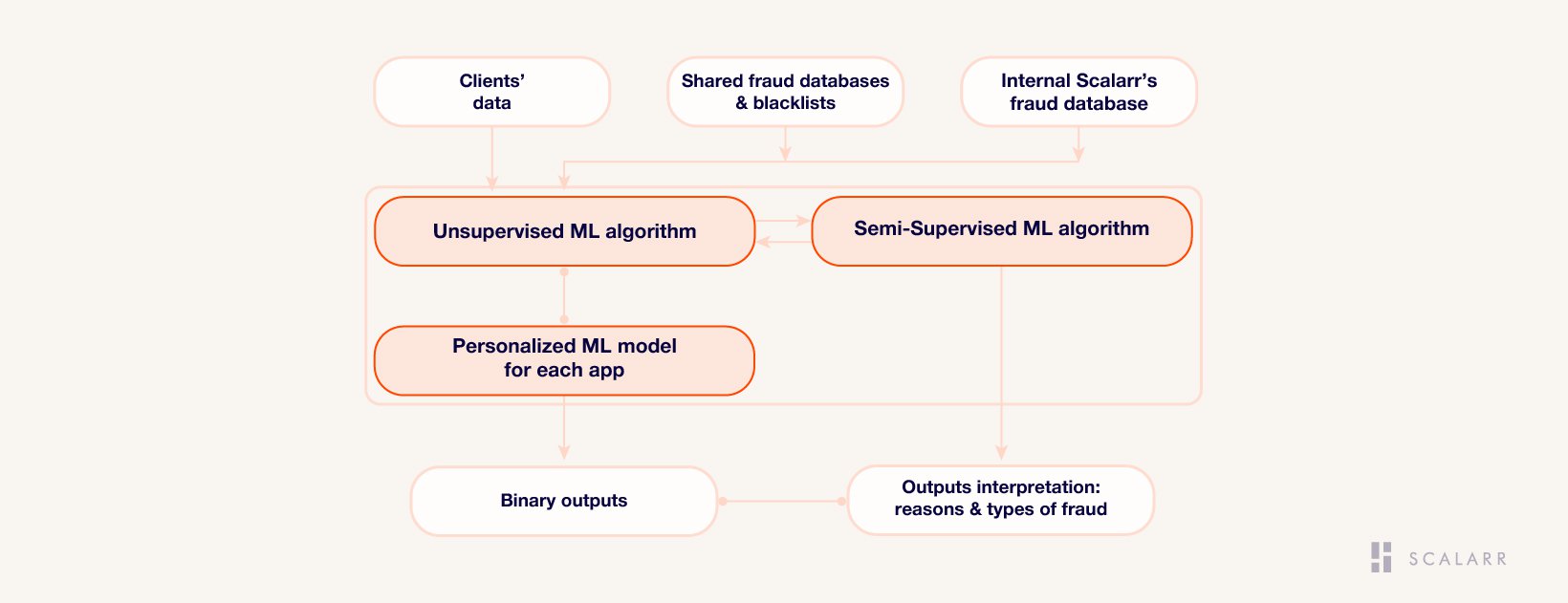
How to fight smart against mobile app-install ad fraud
As we mentioned above, the ML-based identification is showing the highest level of fraud detection among other methodologies. It can find patterns which is not directly obvious to humans. And this moment has a crucial importance, since the total share of the smart bots fraud already prevails over the share of attributive fraud among our clients by 37%.
True ML is a feature of the solution, which continuously learning and generating the personal model of fraud identification for every mobile app. It takes into account all objective and behavioral features and geographical differences. So every client of such solution has his own specific and personal ML algorithm. The presence of such technology is crucial for the highest completeness of fraud detection (identifying all known types of mobile app-install ad fraud) and fraud detection accuracy (the solution’s ability to identify fraud with the minimal level of mistakes).
Machine learning is an excellent candidate to pursue fraud detection in a scalable manner with relatively quick and high-quality results. The aim of ML is to provide an accurate result where rules-based systems would either reject installation, or hold the installation pending a costly manual review. ML-based anti-fraud solution are five to ten times better in fraud detection than rules-based ones.
***
By Scalarr’s estimates, developers lose averagely from 10% to 40% of their marketing budgets, which turns into the number of $6.5 billion of the global economic losses we mentioned in the beginning. And, according to our prediction, fraud rate will grow by 20% in 2018 and become the biggest threat to advertising spend over the next 5 years. So, it’s high time to take up arms against thieves of marketing budgets.
The only solution for mobile advertisers is to partner with anti-fraud tools that make use of powerful and intelligent technologies such as machine...
The road to Scalarr's foundation was paved with challenges and opportunities and in this in-depth conversation, you'll learn the story of Scalarr f...