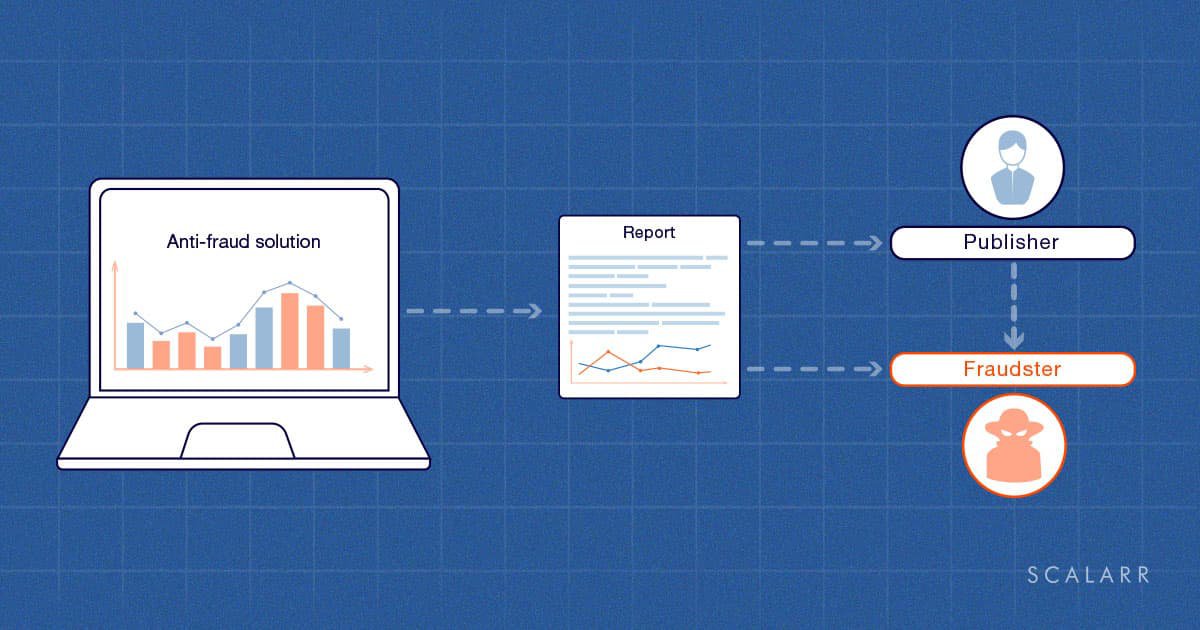
App install ad fraud is constantly mimicking in attempts to get over anti-fraud protection measures. This feature makes this kind of fraud hard to identify and prevent since app developers have to deal with “unnatural”, constantly transforming and unstable properties of the system. Fraudsters make the most of all vulnerabilities of anti-fraud solutions to stay under the radar for as long as possible.
As we mentioned in the recent Scalarr’s report, the global annual losses on the market of app install advertising caused by actions of cybercriminals are about to reach $12,6 billion in 2019. With such a huge potential profit and significant marginality at the same time, fraudsters are not hesitating to invent and employ various tools and channels of reverse engineering. Among them are the following:
1. Independent reverse engineering on actual rejects from anti-fraud solutions.
2. Hacking of anti-fraud solutions.
3. Obtaining insider information from employees of anti-fraud solutions.
4. The use of open information from anti-fraud solutions: support-docs, white papers, articles, reports from conferences, etc.
5. Requesting additional reports by anti-fraud solution clients (mobile apps and games developers) for publishers (ad networks) on the grounds for rejects.
6. Negotiations, communication with publishers (ad networks) and different representatives of anti-fraud solutions.
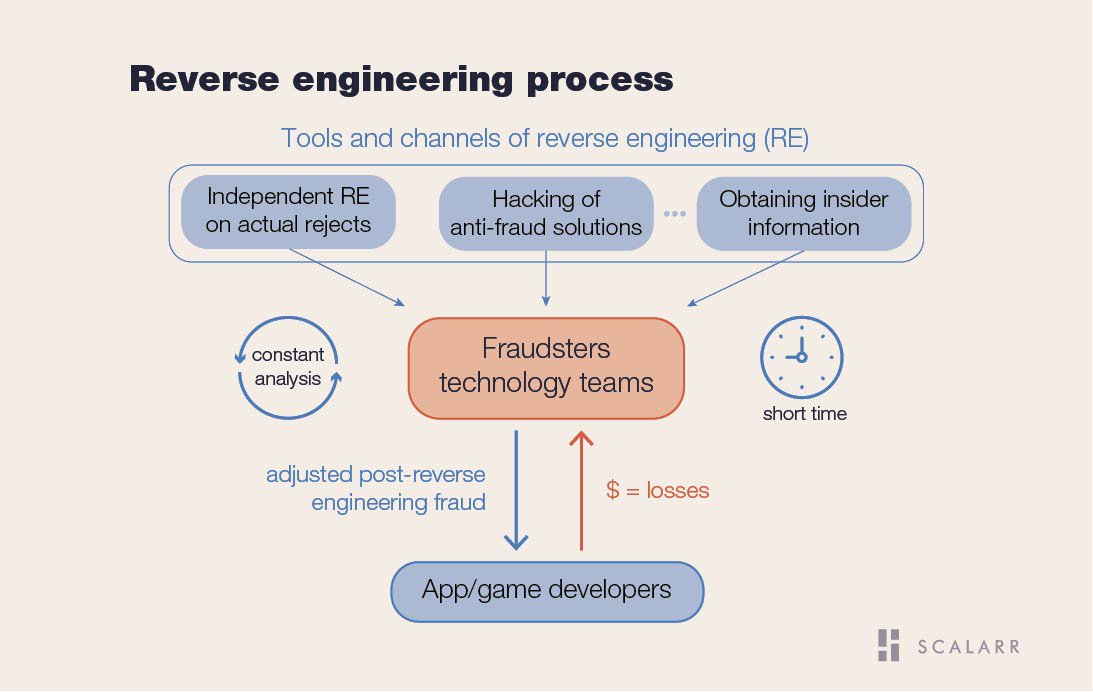
Below are more details on each method of reverse engineering.
- Independent reverse engineering on actual rejects from anti-fraud solutions.
There is almost no protection from such a method of reverse engineering since the operational data of an anti-fraud solution will become available for fraudsters (in the form of standard reports). They receive feedback on which part of their fraud is identified, and which remains undetected. Also, fraudsters can see if there is any effect from their various modifications of fraud.
- Hacking of anti-fraud solutions.
Here we deal with different hacker attacks with an aim to find a weak spot in anti-fraud solution and get access to algorithms and technologies used for fraud identification. This category also includes various DDoS attacks, aimed at causing crashes and shutdowns of anti-fraud systems.
- Obtaining insider information from employees of anti-fraud solutions.
In this case, fraudsters are trying to get the information from employees of an anti-fraud solution by using different means.
- The use of open information from anti-fraud solutions: support-docs, white papers, articles, reports from conferences, etc.
If three previous channels of getting information for reverse engineering were directly based on actions of cybercriminals, then this channel would be completely under control of anti-fraud providers. Unfortunately, many tracking providers in pursuit of greater “interpretability”, open almost all their metrics, algorithms, and rules. With the intention to become more transparent to their clients, they can also reinforce the reverse engineering issue, since all open information sooner or later will come to the hands of criminals. And all this information can be further used for fraud modifications.
With the intention to become more transparent to their clients, anti-fraud solutions can also reinforce the reverse engineering issue, since all open information sooner or later will come to the hands of criminals
- Requesting additional reports by anti-fraud solution clients (mobile apps and games developers) for publishers (ad networks) on the grounds for rejects.
The cooperation between publishers (ad networks) and advertisers (developers of mobile games and apps) implies the fact that they are partners in the first place and advertisers are not able to reject traffic by themselves without explanation.
For example, when it comes to anti-fraud solutions for financial systems, the rejection of fraudulent transactions can be done on an autonomous basis. In this case, an advertiser has to recognize a clear fraud pattern. Otherwise, there is a temptation of unreasonable false-positive allegations of providing fraudulent traffic.
Unfortunately, such practice is widely used by fraudsters, who demand very detailed additional reports from an advertiser with an explanation of why their traffic was labeled as a fraudulent. Besides the information from standard reports, some anti-fraud solutions are also provide that demanded information, which gives a more clear picture for fraudsters of how to overcome protective measures.
- Negotiations, communication with publishers (ad networks) and different representatives of an anti-fraud solution.
Similarly to the situation with the demands of additional reports, fraudsters are also trying to get important information during negotiations and use it later for reverse engineering.
Some more specific examples of reverse engineering
1. An example with the “% of new devices” parameter
Some time ago, one of the anti-fraud solutions has introduced a new metric for identifying bots and device farms - “% of new devices”. This metric was applied to show new devices, that weren’t previously identified by this anti-fraud solution among other apps/games. For developers having quite a big base of devices, this metric allowed to evaluate whether they are dealing with bots/device farms in connection to the percentage of new devices. With the standard percentage of 15-20%, any cohort with about 80-90% of new devices would point at the possible presence of fraudulent activity with resetting device parameters and generating new ones. This metric was publicly introduced and just 2 weeks after, forums of app developers and advertisers became full of questions like “Why does my app have the abnormal peaks of organic installs without any post-install activity afterward?”. Nobody knew the exact answer at that time. But one of the versions - it was reverse-engineering of the “% of new devices” metric made by fraudsters. It was enough for them just to download some more apps organically before downloading the target app, and this fraudulent device no longer had been displayed as a "new" and already had a history of app downloads. So this metric has lost its accuracy in fraud identification.
2. An example with modified click spam
Click spam fraudsters have quickly noticed that anti-fraud solutions identify them through an abnormal TTI (Time To Install) distribution by days. A "long tail" with the TTI of 2,3,4 days was clearly pointing at click spammers. So the next step in this game was made by fraudsters: they have started to simply "cut off a long tail", leaving one day installs only. Using various techniques, modified click spam fraudsters try to limit the TTI of their traffic up to 1 day, thereby hoping to be less visible this way.
Modified click spam can also use new ways to "infect" users. For example, through wi-fi access points in public places. In this case, users click on the elements of the UI start page, and all subsequent organic devices automatically get to click-spammers. Some click spam fraudsters can even modify the attribution type, changing it from click to view for less visibility.
3. An example of faking post-install and financial events
Many advertisers often provide publishers (ad networks) with a list of post-install events and its benchmarks, which can be used for further traffic optimization. Such a list may include the following metrics:
- % of paying users;
- retention rate (Day 1 / Day 7);
- % of registration rate;
- % of ROI (Day 1 / Day 7).
On the one hand, for non-fraudulent publishers such metrics are very helpful in the optimization of different ad campaigns. On the other hand, such information would also be of great help for fraudsters that use smart bots and intelligent device farms. And they would be trying to fake exactly these event benchmarks while staying invisible to the advertiser.
How Scalarr resists reverse engineering
One of the conceptual differences of Scalarr is the maximum level of protection with the minimum disclosure of algorithms and technologies behind the solution.
For instance, let’s take reverse engineering by the means of hacking and obtaining insider information from employees. For such types of attacks, Scalarr undertakes different technical, organizational and legal protection measures.
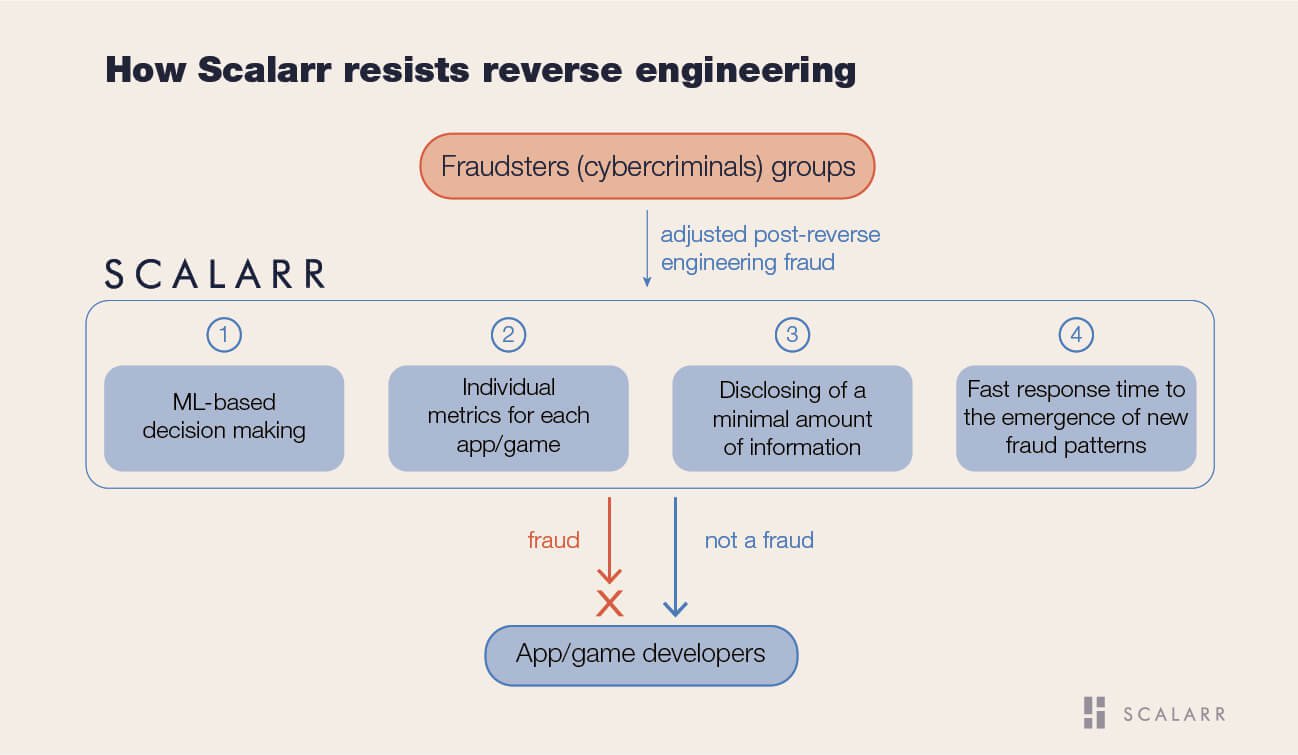
Below we review a fundamentally higher level of protection from reverse engineering and other types of attacks, which consists of four blocks:
1. The concept of Scalarr’s decision making for each install is based on machine learning by employing thousands of data points, hundreds of features and multiple relationships between them. The amount of all these features and data points is not a static number, but a constantly changing “data lake”. In this way, the stability and sustainability of fraud detection are ensured. Even if fraudsters change some metrics in the process of reverse engineering, the system will still detect fraud by using many other features, which remained unchanged. However, many of anti-fraud solutions use simple rules or singled-out metrics for fraud identification. As was described above, such a decision model is extremely unstable when it comes to fraudulent reverse engineering.
2. Decision-making models individually taught for each app also allow to significantly increase the protection level since fraudsters are not able to know all individual metrics and specifics of each app or game. So, their attempts to reverse engineer all apps at once will be immediately detected by the decision-making model. Such an approach has way more advantages in effective fraud identification in comparison to the “average” approach applied by many of solutions, which doesn't take into consideration all the differences between apps or games.
3. Disclosing a very small amount of information, used for decision making. As was mentioned above, the decision is made on the basis of thousands of data points, hundreds of features and multiple relationships between them. Subsequently, additional algorithms mark a few fraud features that can be understood by the human.Only these fraud features are displayed on the Dashboard and come with advertiser’s reports to fraudsters. These fraud features don’t disclose any specific metrics, but only describe the general working principle of a specific algorithm. Similarly, in white papers, articles, and additional reports, Scalarr uncovers a very small fraction of the actual amount of information. At the same time, many anti-fraud solutions open up literally all their metrics and benchmarks, which makes reverse engineering threat just a matter of time.
4. Fast response time to the emergence of new fraud patterns and/or reverse engineering. While larger companies tend to change their algorithms very slowly, here at Scalarr we do this with lightning speed.
Together all these measures allow providing the maximum possible level of protection against reverse engineering.
Final thoughts
Fraudsters are methodically developing more refined schemes and methods to fake installs. In 2018 the fastest mimicry occurred in 18 hours. In this article we reviewed the most common channels of mimicry and provided real examples of how reverse engineering works in the app install ad fraud area. Even though fraudsters continually develop new techniques to imitate real user behavior, Scalarr has proven there are working ways to resist. In general, the fight between fraudsters and anti-fraud solutions is not a battle, but a lasting war, that can be won only by the joint forces of anti-fraud providers and app developers.
The only solution for mobile advertisers is to partner with anti-fraud tools that make use of powerful and intelligent technologies such as machine...
The road to Scalarr's foundation was paved with challenges and opportunities and in this in-depth conversation, you'll learn the story of Scalarr f...