The mobile app companies are more frequently facing new evolving types of fraud they have never seen before. They are already familiar with such common and widespread types of fraud as click injections, classic click spam, and device farms. However, during the course of the current year more and more mobile app companies spending money on allegedly qualitative traffic, which do not bring the expected outcome. Such a case usually indicates coming across more sophisticated types of fraud, which are more problematic to identify. It could be modified click spam, smart bots, organic stealing (soft fraud) or mixed fraud. Every mentioned type of fraud is worth a separate detailed analysis, but in this article, we brought to review a so-called “mixes” or the mixed fraud. Forewarned is forearmed, so let’s see how it works.
Today, mixed fraud is the most advanced type of scam. It usually consists of different fraud types mixed together in one indivisible bundle. But mixed fraud may come out in some even more insidious variations with the “facade” of clean traffic hiding the fraudulent traffic.
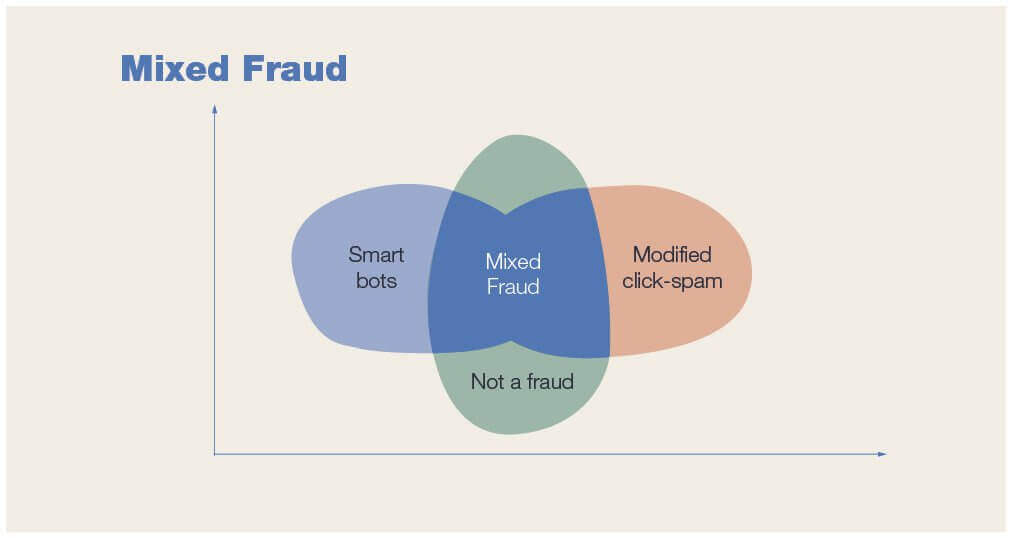
The general principle of “mixes” grounds on the conscious use of several various types of fraud to get over the known protection measures of fraud detection tools. In other cases, it may be also a chaotic mixture of different types of fraud if connected to the publisher with an uncontrolled number of re-brokering levels.
The mixed fraud inside the cohort can be identified in the following combinations:
- One type of fraud and non-fraudulent traffic
- Several types of fraud
- Several types of fraud and non-fraudulent traffic
The main problem with the identification of “mixes” is that almost all anti-fraud solutions and the manual search for fraud include the analysis of the cohorts within the undivided bundle to the maximum detail. And all the rules and metrics for almost all anti-fraud solutions are available only at this level of detail, which makes them not efficient in this case. An example of such a mixed bundle may be the following: app — publisher — sub-publisher — sub-sub-publisher — campaign.
This type of fraud is hardly detectable also because there are no obvious patterns that could be visually recognized and therefore interpreted as fraudulent traffic. So there is still a lot of work to do for the anti-fraud industry in order to be fully prepared for this type of fraud every time it shows up.
However, already today mixed fraud can be identified by employing Machine Learning algorithms. The conscious steps toward fighting mixed fraud especially crucial in the light of growing threat: the traffic analysis outcomes of Scalarr clients make a clear picture, where the “mixes” share make up to 15,3% of all fraudulent installs.
modified click spam” and “smart bots - modified click spam - real users”.
Even despite the issue of proper identification of “mixes”, there is one efficient protective measure, which based on the traffic clustering technology. This technology relies on the ML algorithm, which isolates individual fraudulent clusters inside the indivisible bundle. Below is a review of “mixes” on a few short examples from the two most popular and attractive for fraudsters app categories: Games and e-Commerce.
1. A popular mobile MMORPG game.
A group of fraudsters disguised two types of fraud: smart bots and modified click spam. The idea behind their actions was in programming smart bots with quite short TTI. By doing so, fraudsters made a common, looking similar to organics, TTI distribution for this “smart bot — click spam” mix. In the given situation, a developer without advanced anti-fraud protection would have considered such a bundle (sub-publisher) as absolutely normal and qualitative. For even more confusion mixed fraud was intentionally divided into many small bundles (sub-publishers). Each of these fraudulent clusters was successfully identified thanks to the active use of Unsupervised Machine Learning (by applying to clustering models) by Scalarr. As a result, the mobile game developer was able to see the fraudulent attack, reject the fake installs and save money on them.
2. An e-commerce shopping app.
Here fraudsters were trying to mix click injection fraud with non-fraudulent traffic. The premise of such an attack was in the fact that the “click injection— non-fraudulent traffic” mix makes the overall traffic look like a non-fraudulent. In this case, since we didn’t have fully labeled data sets for such kind of fraud, we took an advantage of new data points from Google Play (such as referrer time, install time, etc.) for the further traffic clusterization. Such an approach allowed us to have fully isolated individual fraudulent clusters inside the indivisible bundle and clearly recognize the fraudulent and the non-fraudulent traffic.
***
At the moment, “mixes” are one of the most problematic types of fraud in terms of identification. And, since, as we mentioned above, mixes account for 15,3% of all fraudulent installs, the effective measures here is a matter of great importance. With quite a low performance shown by the manual search and rule-based solutions in the identification of such type of fraud, we can make a conclusion that today only traffic clustering technology based on true machine learning algorithms allow recognizing the fraudulent and the non-fraudulent traffic, as well as the various types of fraud with high accuracy. So effective protection here is just a matter of responsible approach and right choice.
The only solution for mobile advertisers is to partner with anti-fraud tools that make use of powerful and intelligent technologies such as machine...
The road to Scalarr's foundation was paved with challenges and opportunities and in this in-depth conversation, you'll learn the story of Scalarr f...