When we talk about the fastest-growing app categories, we cannot help noticing shopping apps. This category was on the fourth place in terms of sessions with 25% growth in 2016, but in 2017 it showed 54% growth spurt, which makes shopping apps one of the fastest growing app categories at the moment. Another important change over the last years is that retailers who have both a mobile website and a mobile app generated 67% of their sales from mobile devices. But numbers for the mobile web and mobile apps are not equal: mobile apps account for 44% of e-commerce transactions as compared to 23% for the mobile web in 2017. Also, the conversion rate on shopping apps is more than 3 times higher than on the mobile web. That puts shopping apps, for which the CPI is 35% higher than the average, at high risk for the mobile ad fraud.
According to Scalarr’s estimates, shopping apps along with mobile games and business & finance apps are the most attractive categories for fraudsters attacks. The shopping apps category itself is notable for a high percentage of such fraud types as bots, smart bots, classic and modified click spam, and, more recently, the various types of mixed fraud. These types of fraud without a proper protection can greatly affect any shopping app, causing a decrease in the revenue growth. Fraudsters harm all financial indicators, which can eventually lead to the adoption of wrong decisions because they will be based not only on the data of real users but also on the behavior of the fraudsters. In order to see how app-install fraud influences the shopping apps category, let’s dive into Joom experience of dealing with the app install fraud.
Joom fights fraud with Scalarr's Machine Learning Algorithms
Joom is a popular platform for e-commerce with an easy-to-use app where you can buy more than 4 000 000 products, all from verified sellers. By installing the app users will be able to browse a huge catalog of products that covers everything from clothing to kitchenware or electronics. Joom was recently included to the TOP 5 Most downloaded apps in Online Deals category. At the moment Joom’s UA team successfully buys traffic in the CIS countries and Europe and also plans to enter the US market soon. While actively expanding into new markets, they were facing app install fraud all the way down, so now they are quite familiar with the fraud issue. Since February 2018 Joom have been using the anti-fraud solution, based on the machine learning algorithms from Scalarr.
The integration with Scalarr has disclosed some important data to Joom user acquisition team. For the analyzed period, the number of identified fraudulent installs has exceeded 947K. The causes for those almost a million fraudulent installs were divided into three groups as shown in the diagram below:
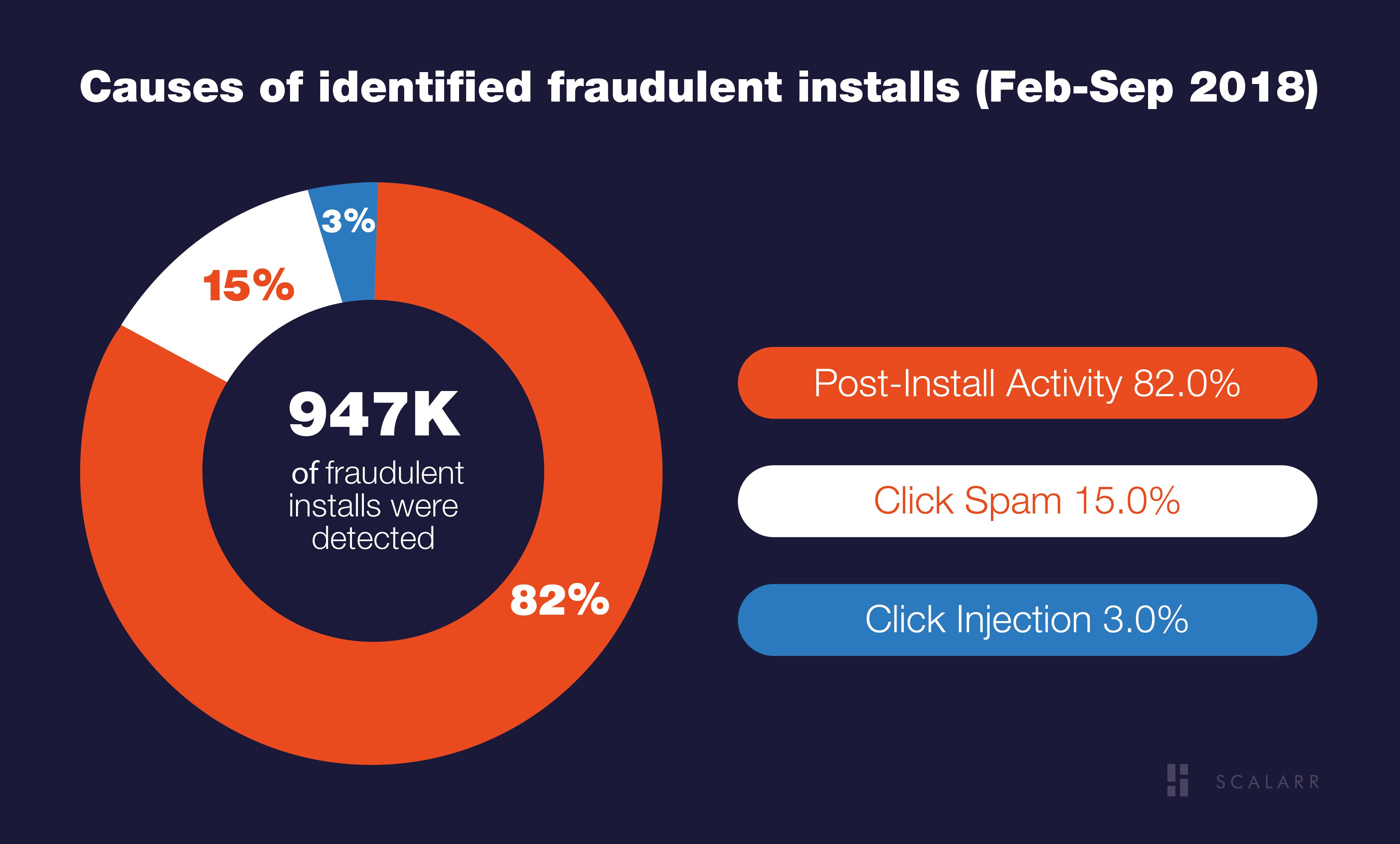
Among Click Spam just 10% of conversions were marked as Classic Click Spam and 90% conversions were marked as Modified Click Spam. Both Click-Spam fraud types are inherently organic, so all financial indicators, post-install events, other attributes of the device and install are absolutely real. A “long tail” with the TTI of 2,3,4 days was clearly pointing at click-spammers. And they have modified their tactics to “cut off” the long tail, leaving visible one day installs only. Thus, Modified Click Spam becomes more difficult to identify.
As shown above, the biggest part of fraudulent installs (82%) fall into the fraudulent post-install category. A deeper analysis of this category has indicated 63% of conversions as Classic bots (without post-events) and 37% of conversions as Smart bots blended together. The latter kind of fraud is called “smart” because of its ability to fully emulate the user behavior by performing all post-install activities for a long period. From a human perspective, smart bots look almost alike real users by having the personal IP, device ID, etc. Scalarr detects the smart bot fraud and blends or ‘mixes’ of different types of fraud in a number of ways, but its workhorse method uses unsupervised machine-learning (UML), which basically looks for the clusters of abnormalities, and Semi-Supervised models trained on past examples of confirmed good and fraudulent behavior. Because data is extremely unbalanced and highly dimensional, Scalarr uses both approaches to be able to validate and compare the outputs. Thus, the models’ performance is measured at the rate of uncovering fraudulent versus good installs at various data points thresholds looking for strong deviations in the first model. And for the latter, these data points are encoded in its features as a kind of weighted logical disjunction between true-positive and false-negative rates. That gives a lot of opportunities from dramatically increased accuracy in identifying the exact fraudulent patterns to the ability of detecting a totally new unknown and entirely modified ones.
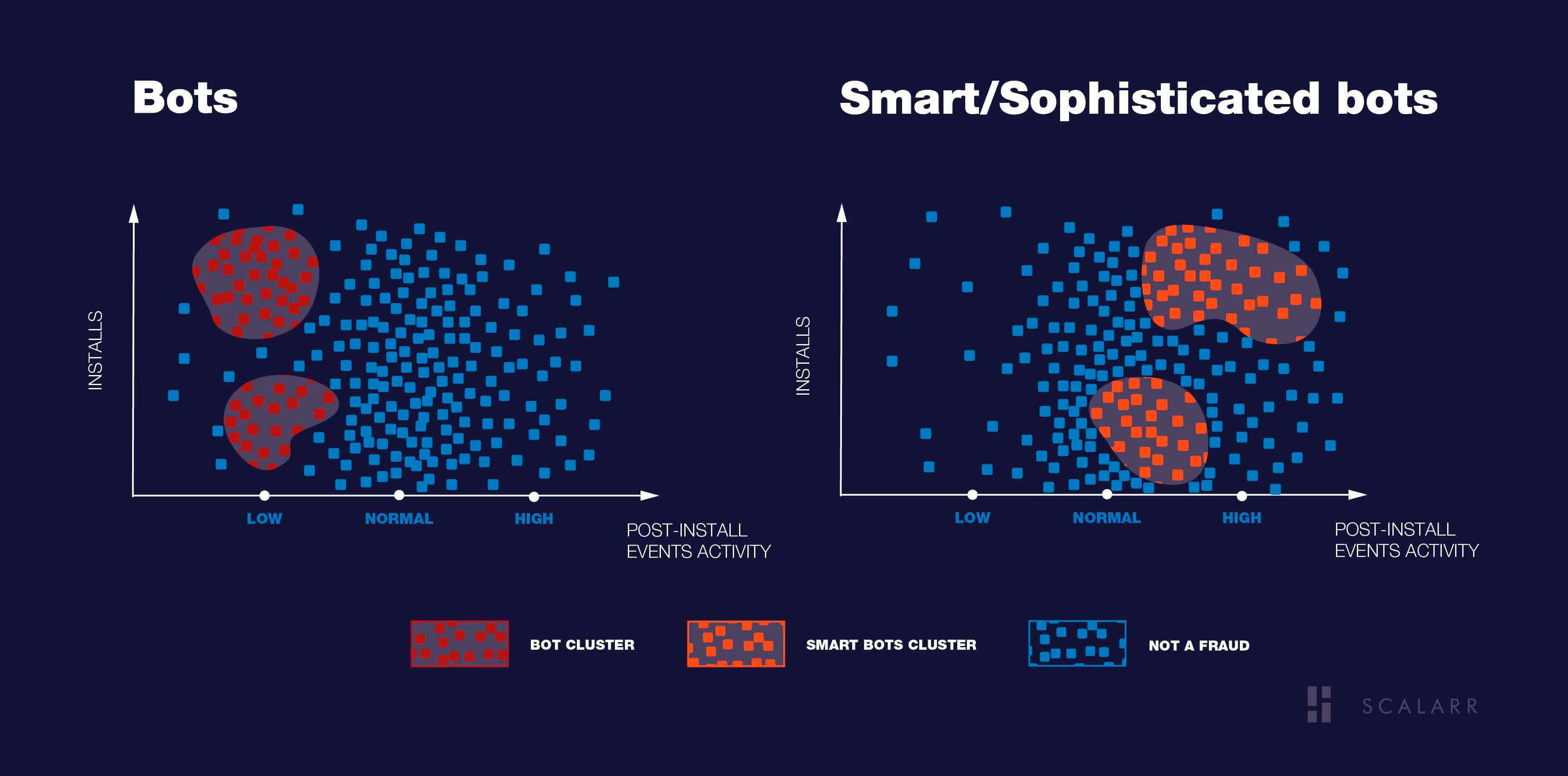
On Android, the “primitive” smart bots were prevalent with a high percentage of users who completed the events. Along with it, the ratio of the main event to the “users” who completed this event was 1:1, still that was not the only one abnormality, which made Scalarr solution mark the installs as fraudulent. Fraudsters incubate their accounts for a while with some payments at minimum rates done to encrypt the nature of their complicated logic and make the bunch looking naturally. Still, these clusters of abnormal events variables can be seen via rightly computed analysis.
Interesting, that the share of mixed fraud and click-injections was insignificant. But beginning from the third month of analysis, the mixed fraud began to dominate in the traffic for the Android version of the app. The nature of fraud has also undergone several changes in the iOS version of the app: the share of click spam has decreased significantly. Bots began to dominate.
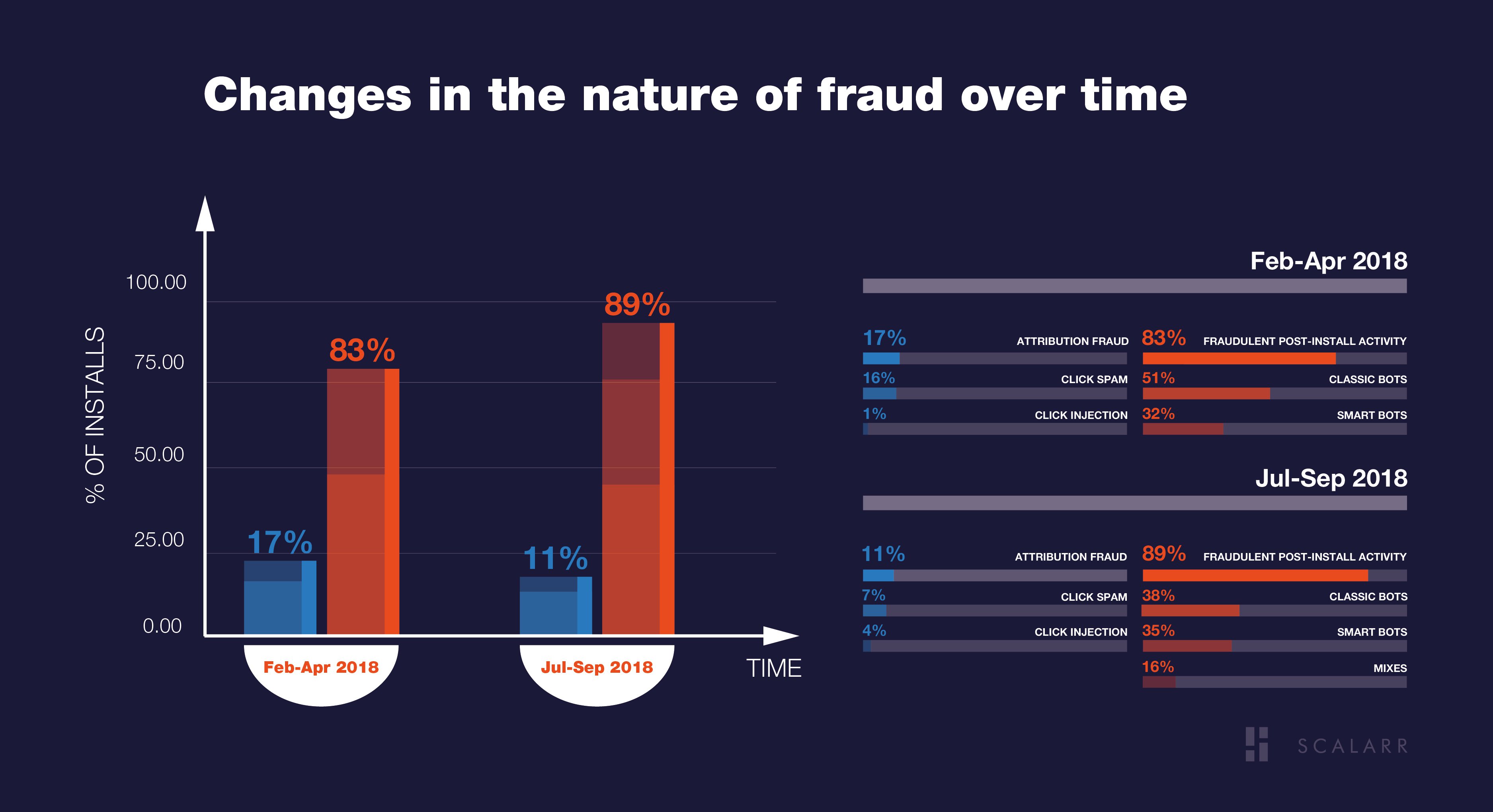
As a result, during the past eight months of fraud detection was discovered a huge amount of unwanted activity. However, all these fraudulent attacks took place not at once, but more in “the storm after the calm” manner. Knowing the fact that the fraud is a cyclical phenomenon, we should always be on alert: as soon as it seems that there is no fraud threat anymore, the fraudsters start to attack with renewed force, changing and adapting their tactics to the detection rules that was used before. Joom experience has proved it. And now Joom along with a big amount of other mobile app companies consider the Machine Learning as a must-have tool in their everyday work.
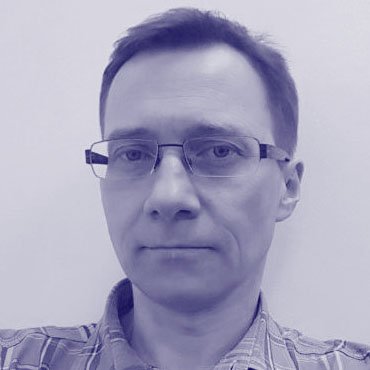
Behind the Scenes
Mobile ad fraud is a serious problem which undermines the mobile advertising industry. The fraudsters change their algorithms very quickly, implementing new ways of fraud attacks and incubating their accounts for a long time to make them look natural. The losses due to the fraud are increasing constantly, generally speaking, they are skyrocketing, while traditional fraud detection approaches remain mostly ineffective, especially for retailers, where the cost of fraud goes even higher due to very high costs of customer acquisition and management.
Scalarr implements a few layers of machine learning to detect the mobile app install fraud, such as unsupervised and semi-supervised models. The recent research comes with the neural network models implemented into production aiming to catch the whole crime ring, connecting the bots and botnet networks as well as installs generated by the same smart device farms through the number of publishers. Still, neural networks can be used for different fraud issues, which we will address in our next articles for reading.
Meanwhile, you can read more about the different types of mobile app install ad fraud in Scalarr’s report.
The only solution for mobile advertisers is to partner with anti-fraud tools that make use of powerful and intelligent technologies such as machine...
The road to Scalarr's foundation was paved with challenges and opportunities and in this in-depth conversation, you'll learn the story of Scalarr f...